Libratus Poker Ai
The blueprints for Libratus – the poker AI bot that crushed professional players in a Texas hold ’em tournament earlier this year – were published on Monday in a research paper. The software's victory over humans sparked a lot of headlines as it demonstrated a computer mastering an imperfect information game. Libratus: the world's best poker player I n January 2017, four world-class poker players engaged in a three-week battle of heads-up no-limit Texas hold ’em. They were not competing against each other.
Libratus, the artificial intelligence (AI) engine designed by Professor Tuomas Sandholm at Carnegie Mellon University (CMU) and his graduate student Noam Brown has made an impression on Jason Les, one of the world’s top poker players. Poker News, the poker industry’s online news magazine, recently interviewed Les. A couple questions were telling when asked about which is a better name for his firstborn child and which is the more annoying opponent, Claudico or Libratus. For both questions, he responded with Libratus.[1],[2]
In January, Les and three others of the world’s top four poker champions—Dong Kim, Daniel McAulay, and Jimmy Chou—were challenged to 20 days of No-limit Heads-up Texas Hold ‘em poker at the Brains versus Artificial Intelligence tournament in Pittsburgh’s Rivers Casino. Libratus beat all four opponents, taking away more than $1.7 million in chips.
Les also considered that being selected to play against Libratus as his proudest poker accomplishment to date.
During the tournament, Dong Kim said about Libratus, “I didn’t realize how good it was until today. I felt like I was playing against someone who was cheating, like it could see my cards. I’m not accusing it of cheating. It was just that good.”[3] Kim also noted during the tournament that “he and his fellow humans have no real chance of winning.”[4]
Texas Hold ‘em, The Holy Grail
“In the area of game theory,” said Sandholm, “in which I’ve been working since 1989, No-Limit Heads-Up Texas Hold ‘em is the holy grail of imperfect-information games.” According to Sandholm, this version of poker was really the last game that had not been cracked by AI, in the sense of becoming better than humans. AI has already beaten top experts of other games, such as chess and go, but Texas Hold ‘em is different. In those games, the game play is open. All players know about where the pieces are at any time and the strategic possibilities presented, and thus can directly solve endgame strategies. In Texas Hold ‘em, players have a limited amount of information available about the opponents’ cards because some cards are played on the table and some are held privately in each player’s hand. The private cards represent hidden information that each player uses to devise their strategy. Thus, it is reasonable to assume that each player’s strategy is rational and designed to win the greatest amount of chips.
“Besides being a limited information play, the stakes space is huge. There are 10160 different situations the player can face. You can’t solve all those possibilities, and you can’t solve a sub-game of the game tree with information from a sub game only. How you play a sub-game actually depends on how you play in totally different parts of the game. It takes totally different algorithms from a game like chess, checkers, or go.”
Learning and Reasoning in a Limited Information Environment
Libratus is an AI system designed to learn in a limited information environment. It consists of three modules:
- A module that uses game-theoretic reasoning, just with the rules of the game as input, ahead of the actual game, to compute a blueprint strategy for playing the game. According to Sandholm, “It uses Nash equilibrium approximation, but with a new version of the Monte Carlo counterfactual regret minimization (MCCRM) algorithm that makes the MCCRM faster, and it also mitigates the issue of involving imperfect recall abstraction.”
- An subgame solver that recalculates strategy on the fly so the software can refine its blueprint strategy with each move.
- A post-play analyzer that reviews the opponent’s plays that exploited holes in Libratus’s strategies. “Then, Libratus re-computes a more refined strategy for those parts of the stage space, essentially finding and fixing the holes in its strategy to play even closer to Nash equilibrium in those spots,” stated Sandholm.
From Claudico to Libratus
Les and Kim also played against Sandholm’s previous AI poker player, Claudico, in 2015. And, while the two programs were authored by the same persons, Libratus is a different application. “Between Claudico and Libratus, we developed a better equilibrium-finding algorithm for module one, so we could do much finer-grained abstractions. The endgame solver of module two is a big improvement. In Claudico, we found during the 2015 tournament, that the application ran well with or without the endgame solver we had built. Libratus’ endgame solver improves play between 80 to 100 milli-big blinds per hand (mbb/hand),[5] increases safety, or non-exploitability, and it is a nested endgame solver, whereas Claudico was not. In module three, Claudico essentially continued overnight what it did in module one, while in Libratus, it actually fixes exploitable holes in its own strategy. That’s an entirely new aspect,” explained Sandholm.
Sandholm and his students have been working on poker optimization for 13 years. He typically had two PhD Students working with him, “but the actual code for Libratus was written from scratch,” stated Sandholm. He and his student, Noam Brown, spent a little over a year writing Libratus. “In 2015, we couldn’t beat the best players with Claudico, and many wondered how much we could accomplish in just a little over a year of work on Libratus,” added Sandholm. “In fact, the international betting sites were putting us at from four to five to one odds against.” It turned out not to be a safe bet.
Bridges, the Supercomputing Poker Machine
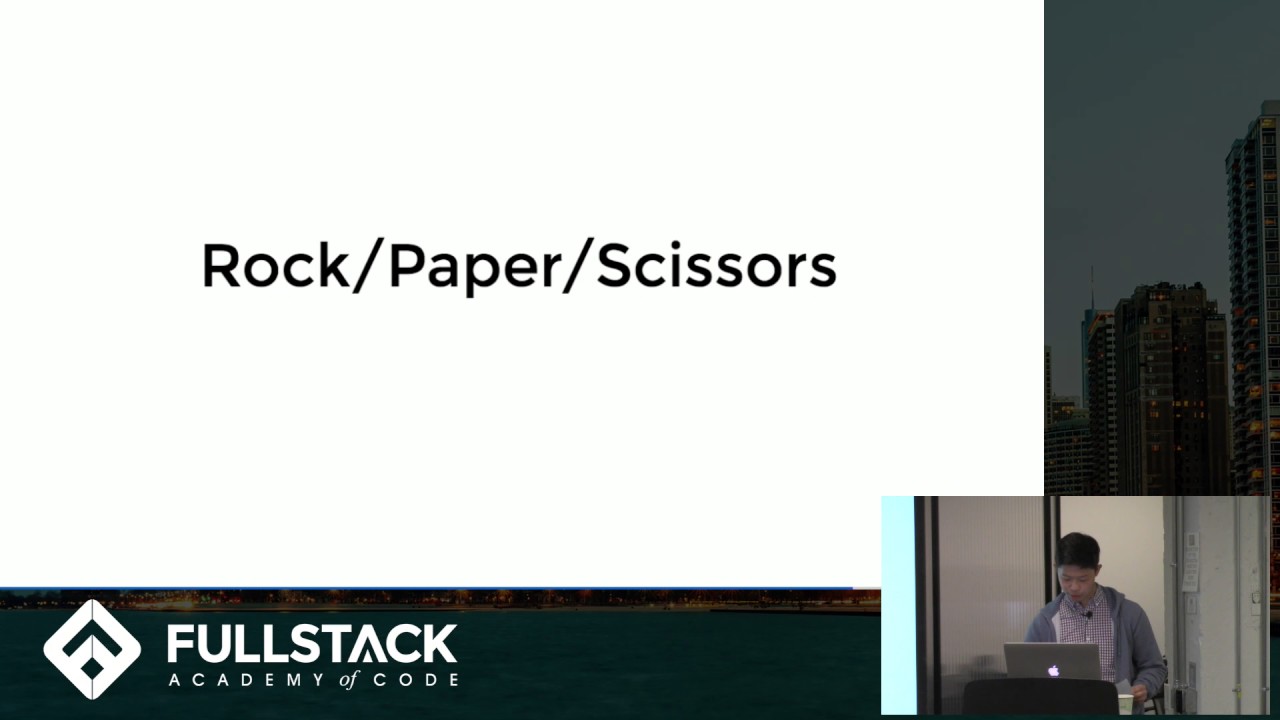
For the tournament, Libratus ran on the newest supercomputer, called Bridges, at the Pittsburgh Supercomputing Center (PSC) The system is a unique configuration built for traditional types of applications, like simulating astrophysical phenomena, and for machine learning workloads, like Libratus. Bridges was built by Hewlett Packard Enterprise (HPE), using Intel® Xeon® processors and Intel® Omni-Path Architecture (Intel® OPA), a new networking technology from Intel that is now being used in the world’s fastest supercomputers. Bridges comprises 846 nodes of computing power, with both “regular memory” nodes and “high-memory” nodes, the latter often used in. WIRED. Retrieved 2017-01-24.
[4] Ibid.
[5] Mbb/g is a normalizing measure of well a player plays.
Libratus Poker Aircraft
# # #
This article was produced as part of Intel’s High Performance Computing (HPC) editorial program, with the goal of highlighting cutting-edge science, research and innovation driven by the HPC community through advanced technology. The publisher of the content has final editing rights and determines what articles are published.
An artificial intelligence called Libratus beat four top professional poker players in No-Limit Texas Hold’em by breaking the game into smaller, more manageable parts and adjusting its strategy as play progressed during the competition, researchers report.
In a new paper in Science, Tuomas Sandholm, professor of computer science at Carnegie Mellon University, and Noam Brown, a PhD student in the computer science department, detail how their AI achieved superhuman performance in a game with more decision points than atoms in the universe.
AI programs have defeated top humans in checkers, chess, and Go—all challenging games, but ones in which both players know the exact state of the game at all times. Poker players, by contrast, contend with hidden information: what cards their opponents hold and whether an opponent is bluffing.
Imperfect information
In a 20-day competition involving 120,000 hands this past January at Pittsburgh’s Rivers Casino, Libratus became the first AI to defeat top human players at Head’s-Up, No-Limit Texas Hold’em—the primary benchmark and longstanding challenge problem for imperfect-information game-solving by AIs.
Libratus beat each of the players individually in the two-player game and collectively amassed more than $1.8 million in chips. Measured in milli-big blinds per hand (mbb/hand), a standard used by imperfect-information game AI researchers, Libratus decisively defeated the humans by 147 mmb/hand. In poker lingo, this is 14.7 big blinds per game.
“The techniques in Libratus do not use expert domain knowledge or human data and are not specific to poker,” Sandholm and Brown write in the paper. “Thus, they apply to a host of imperfect-information games.”
Such hidden information is ubiquitous in real-world strategic interactions, they note, including business negotiation, cybersecurity, finance, strategic pricing, and military applications.
Three modules
Libratus includes three main modules, the first of which computes an abstraction of the game that is smaller and easier to solve than by considering all 10161 (the number 1 followed by 161 zeroes) possible decision points in the game. It then creates its own detailed strategy for the early rounds of Texas Hold’em and a coarse strategy for the later rounds. This strategy is called the blueprint strategy.
One example of these abstractions in poker is grouping similar hands together and treating them identically.
“Intuitively, there is little difference between a king-high flush and a queen-high flush,” Brown says. “Treating those hands as identical reduces the complexity of the game and, thus, makes it computationally easier.” In the same vein, similar bet sizes also can be grouped together.
In the final rounds of the game, however, a second module constructs a new, finer-grained abstraction based on the state of play. It also computes a strategy for this subgame in real-time that balances strategies across different subgames using the blueprint strategy for guidance—something that needs to be done to achieve safe subgame solving. During the January competition, Libratus performed this computation using the Pittsburgh Supercomputing Center’s Bridges computer.
When an opponent makes a move that is not in the abstraction, the module computes a solution to this subgame that includes the opponent’s move. Sandholm and Brown call this “nested subgame solving.” DeepStack, an AI created by the University of Alberta to play Heads-Up, No-Limit Texas Hold’em, also includes a similar algorithm, called continual re-solving. DeepStack has yet to be tested against top professional players, however.
How artificial intelligence can teach itself slang
The third module is designed to improve the blueprint strategy as competition proceeds. Typically, Sandholm says, AIs use machine learning to find mistakes in the opponent’s strategy and exploit them. But that also opens the AI to exploitation if the opponent shifts strategy. Instead, Libratus’ self-improver module analyzes opponents’ bet sizes to detect potential holes in Libratus’ blueprint strategy. Libratus then adds these missing decision branches, computes strategies for them, and adds them to the blueprint.

AI vs. AI
In addition to beating the human pros, researchers evaluated Libratus against the best prior poker AIs. These included Baby Tartanian8, a bot developed by Sandholm and Brown that won the 2016 Annual Computer Poker Competition held in conjunction with the Association for the Advancement of Artificial Intelligence Annual Conference.
Whereas Baby Tartanian8 beat the next two strongest AIs in the competition by 12 (plus/minus 10) mbb/hand and 24 (plus/minus 20) mbb/hand, Libratus bested Baby Tartanian8 by 63 (plus/minus 28) mbb/hand. DeepStack has not been tested against other AIs, the authors note.
“The techniques that we developed are largely domain independent and can thus be applied to other strategic imperfect-information interactions, including nonrecreational applications,” Sandholm and Brown conclude. “Due to the ubiquity of hidden information in real-world strategic interactions, we believe the paradigm introduced in Libratus will be critical to the future growth and widespread application of AI.”
Libratus Poker Air 2
To spur innovation, teach A.I. to find analogies
Libratus Poker Aiken
The technology has been exclusively licensed to Strategic Machine Inc., a company Sandholm founded to apply strategic reasoning technologies to many different applications.
The National Science Foundation and the Army Research Office supported this research.
Libratus Poker Air
Source: Carnegie Mellon University